Piecing together different pieces of technology can have a multiplicative effect. I think that’s what happened with this demo: we paired Wi-Fi locationing, low cost hardware, Golioth Pipelines, and n8n (an API workflow tool) to create a “geofence”.
A geofence is a virtual perimeter used to set up alerts or take actions once a device moves outside that virtual perimeter. The example we gave in the video is if you had a tracker on your cat and you wanted to take an action once the device was outside a particular area.
Hardware
The reason we’re calling this a “$2 geofence” is because it’s enabled by the ESP32-C3, a low cost module from Espressif. We put this on the Aludel Elixir as a backup connectivity method if we were again at a conference with no LTE-M coverage.
The ESP-AT firmware does what it sounds like it should do: it responds to AT commands from other microcontrollers talking to it over serial (as many cellular modules also do). One key enhancement is that the ESP-AT mode already works as a connectivity method; in fact, we utilize the ESP-AT firmware as an offloaded Wi-Fi modem when we build and test for the nRF52840 in our Continuously Verified Hardware. In Zephyr, there is an option for utilizing the ESP-AT modem as the main offloaded Wi-Fi modem. This makes it ‘invisible’ to the Zephyr program and acts like any other network interface, since it is built on top of the Wi-Fi subsystem in Zephyr.
One change that was required is we had to re-write how we pulled the information off the ESP-AT modem. Normally the wifi scan
shell command returns the (human readable) names and signal strengths of all the access points (APs) visible to the modem. Instead, we want mac address and signal strength, as that’s what’s expected by the API service we’ll describe below.
Golioth Pipeline
We start by scanning Wi-Fi APs and the tower that the cell modem is connected to. Then we publish that on the Stream service up to the Golioth cloud. Because we’re publishing to a specific topic (instead of my normal, generic default of “sensor”), we can start to peel off that data and send it somewhere interesting. How? With pipelines, of course!
I set up the pipeline to watch on the path wifi_lte_loc_req
(a name of my own making, this could be any arbitrary name). That data gets sent out to a webhook going to n8n. Webhooks more broadly are a generic way to interface between a lot of cloud services, but we use it to send data into the api platform.
n8n
Now that the data is being sent into n8n (a self hosted instance, no less!) we can start doing interesting things with it. This is an area that is full of similar offerings, sometimes specifically targeted at IoT, and other time targeted a business workflows:
If you’re newer to working with APIs and tying stuff together, it might take a bit of time to figure out how queries should be structured and how your setup should respond when there are errors.
API service
We send data from the device to Golioth already formatted for what the location service API service expects. This is not required in the slightest, as Golioth’s Pipelines can morph and transform data to meet the needs of the endpoint. But…why not? It kind of makes sense to have the device publish data in a format that matches the target API service. Then later if we decide to re-target an alternative service, we can use transformations to mold the incoming data to what that new service expects.
For this demo, I’m using the here.com API service. I like that it combines LTE tower + WiFi AP for its API, which means it will lean on whichever provides a more accurate reading (normally Wi-Fi). Again, this service is one of many! There are a range of API services because this is something that phones are often using to determine location from apps.
Once we receive the lat
, lon
, and accuracy
, we actually pass the data back to the device using LightDB State. This two-sided database is a good defacto way to send arbitrary data from the cloud to the device. In the case of n8n, we’re pulling through the original project name, device identifier, and then publishing to the Golioth REST API. This makes it a data “round trip” from device to cloud and back down to device.
Logic and alerts
Since the data is already on the cloud in an API marketplace like n8n…why not use that data to do some cloud side processing? In this case, I wanted to set up a geofence to show that we can trigger logic and alerts on the cloud and even call 3rd party APIs like Slack and Twilio.
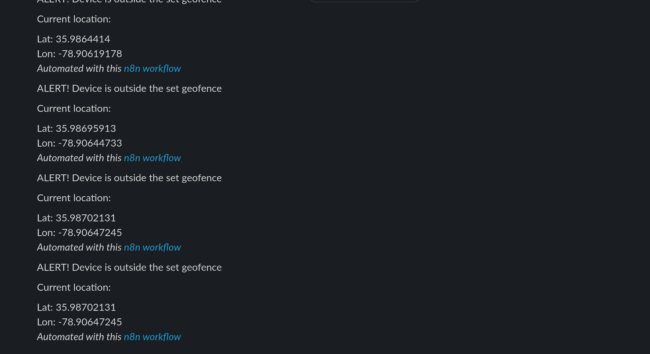
Geofence alert messages being sent into Slack
I asked ChatGPT to help me out with some javascript that would help calculate a true/false output so that I could use that to trigger downstream logic. We insert the lat
/lon
data that was returned from here.com into this algorithm and it pops out whether or not we are inside the “fence”. As of this writing, I am still using a fixed location for where the center of the “fence” is located, as well as the radius of said “fence”. I’m certain it’s possible in n8n or other tools, perhaps as another Webhook or a configurable variable.
Future demos
Hopefully one thing you noticed from this demo is just how much can be enabled with Golioth’s pipelines. Since Golioth takes care of reliably delivering your data to the cloud, the rest is really a matter of configuration. It’s also difficult to know all the different APIs that could be utilized out in the world. Pulling these elements together shows how a hardware or firmware engineer could enact complex device and business logic to create interesting applications out in the real world. If you need any help getting your next project off the ground, stop by our forum!